How do deep neural networks work? Statistical physics meets deep learning in an article published in Nature Machine Intelligence by a researcher from the team “Statistical physics and inference for biology”.
A team of young researchers coordinated by Dr Pietro Rotondo (University of Parma) and including Dr Mauro Pastore (LPENS/LPTMS) has recently proposed an effective theory capable of predicting the performance achieved by a class of deep (“fully connected”) neural networks.
These models process the input they receive, in the form of high-dimensional arrays (for example, images), via successive layers of linear (multiplication by matrices of “weights”) and non-linear (element-wise application of opportune “activation functions”) transformations, to produce a low-dimensional output (such as labels classifying images). A desired input-output rule is implemented by tuning the weights to fit the labelled examples in a given training set, and the effectiveness of the model is assessed by evaluating its generalisation performance, that is its ability to correctly predict input-output associations on previously unseen examples.
In particular, the proposed theory is able to determine the generalisation ability of the network from its fundamental parameters (the width of the inner layers – controlling the number of weights in each of them, the activation function, etc.) and the data used to train it.
This discovery helps to bridge the gap between the theory and practical applications of artificial intelligence, paving the way for the exploration of increasingly complex systems that are closer and closer to those we use every day.
The collaboration includes researchers from several French and Italian institutions, with the fundamental contribution of two young researchers, Rosalba Pacelli (PoliTo and UniBocconi) and Sebastiano Ariosto (Uninsubria), who will defend their PhD thesis soon.
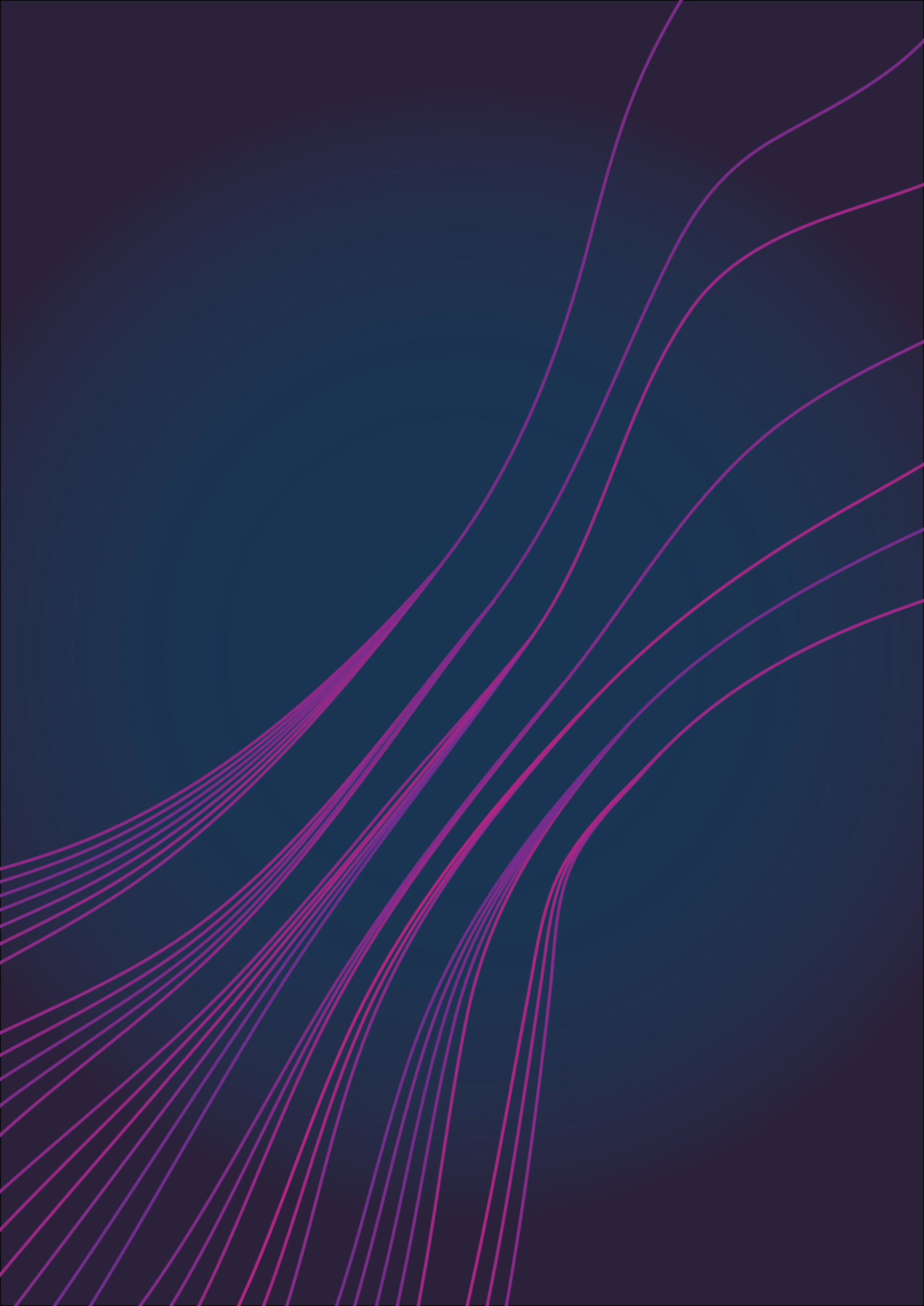
Colors of a deep neural network – Parameters from a trained ResNet-18 model as abstract lines, shaded according to their numerical value. The multitude of lines that here symbolizes an infinite network sparsify to become a finite structure.
More:
https://www.nature.com/articles/s42256-023-00767-6
Affiliation author:
Laboratoire de physique de L’École normale supérieure (LPENS, ENS Paris/CNRS/Sorbonne Université/Université de Paris)
Corresponding author : Mauro Pastore
Communication contact: Communication team